It’s fairly well known that higher education admissions practices have made headlines in recent years, and issues of access and equity have been at the heart of the controversies. In 2019, a highly-publicized admissions scandal known as Operation Varsity Blues revealed conspiracies committed by more than 30 affluent parents, many in the entertainment industry, offering bribes to influence undergraduate admissions decisions at elite California universities. The scandal was not limited to misguided actions of wealthy, overzealous parents, however, and it included investigations into the coaches and higher education admissions officials who were complicit (Greenspan, 2019).
Harvard University has also seen its fair share of scandals including a bribery scheme of its own and controversy over racial bias in the admissions process. In 2019, a group of Harvard students organizing under the title “Students for Fair Admissions” went to court over several core claims:
- That Harvard had intentionally discriminated against Asian-Americans
- That Harvard had used race as a predominant factor in admissions decisions
- That Harvard had used racial balancing and considered the race of applicants without first exhausting race-neutral alternatives.
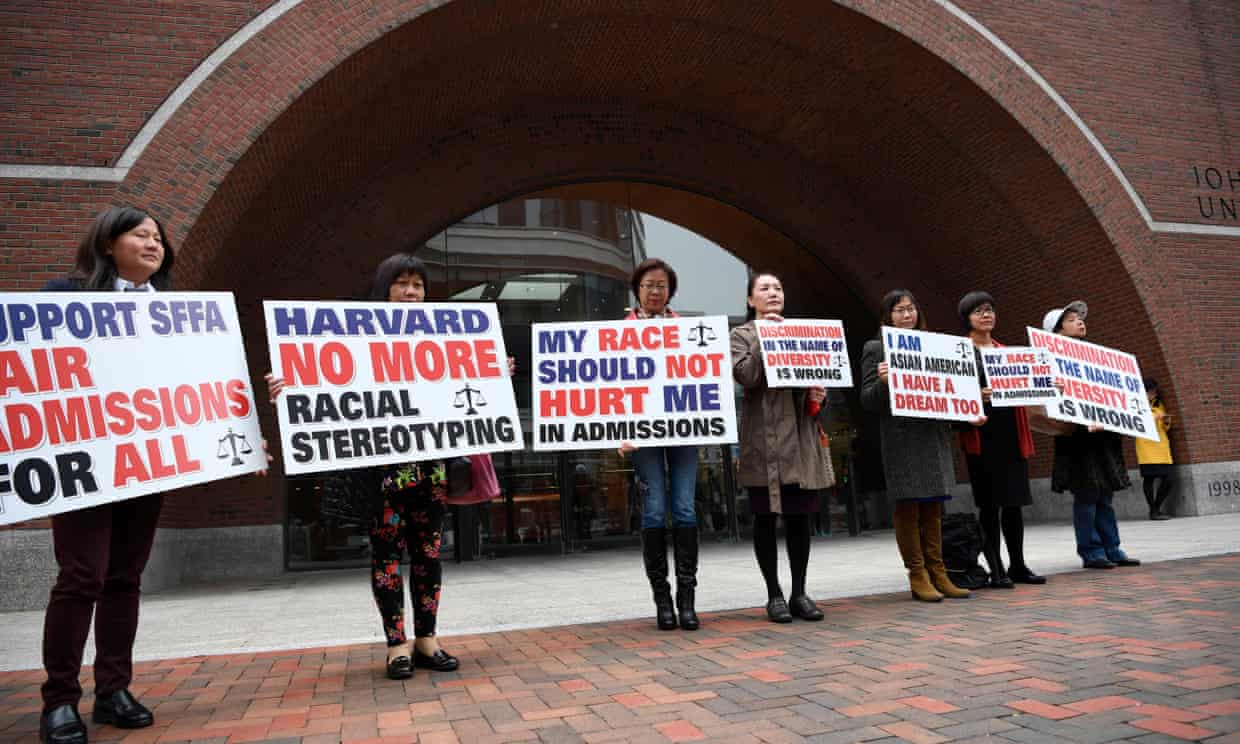
In line with the tenants of affirmative action, the court eventually ruled that Harvard could continue considering race in its admissions process in pursuit of a diverse class, and that race had never (illegally) been used to “punish” an Asian-American student in the review process (Hassan, 2019). Yet regardless of the ruling, Harvard was forced to look long and hard at its admissions processes and to meaningfully consider where implicit bias might be negatively affecting admissions decisions.
Another area of bias that has been identified in the college admissions system nationwide is the use of standardized tests, especially the SAT or ACT for undergraduate admissions and the GRE or GMAT for graduate admissions. Changes in demand for these tests have only accelerated during the pandemic with many colleges and universities making SAT/ACT or GRE/GMAT scores optional for admission in 2020-2021 (Koenig, 2020). Research has oft-revealed how racial bias affects test design, assessment, and performance on these standardized exams, thus bringing biased data into the admissions process to begin with (Choi, 2020).
That said, admissions portfolios without standardized test scores have one less “objective” data point to consider in the admissions process, putting more weight on other more subjective pieces of an application (essays, recommendations, interviews, etc.). Most university admissions processes in the U.S.—both undergraduate and graduate—are human-centered and involve a “holistic review” of application materials (Alvero et al, 2020). A study by Alvero et al exploring bias in admissions essay reviews found that applicant demographic characteristics (namely gender and household income) were inferred by reviewers with a high level accuracy, opening the door for biased conclusions drawn from the essay within a holistic review system (Alvero et al, 2020).
So the question remains—how do higher education institutions (HEIs) implement equitable, bias-free, admissions processes that guarantee access to all qualified students and prioritize diverse student bodies? To assist in this worthwhile quest for equity, many HEIs are turning to algorithms and AI to see what they have to offer.
Lending a Helping Hand
Without the wide recruiting net and public funding that large State institutions enjoy, the search for equitable recruiting/admissions practices and diverse classes may be hardest for small universities (Mintz, 2020). Taylor University—a small, private liberal arts university in Indiana—has turned to the Salesforce Education Cloud (and the AI and algorithmic tools within) for assistance in many aspects of the admissions and recruiting process. The Education Cloud and other similar platforms “…use games, web tracking and machine learning systems to capture and process more and more student data, then convert qualitative inputs into quantitative outcomes” (Koenig, 2020).
As a smaller university with limited resources, the Education Cloud helps Taylor’s admissions officers zero-in on the type of applicants they feel are most likely to enroll, and then identify target populations that exhibit similar data sets in other areas of the country based on that data. Taylor can then strategically and economically make recruiting efforts where they’re—statistically speaking—likely to get the most interest. With fall 2015 boasting their largest Freshman class ever, Taylor is, in many ways, a success story, and Taylor now uses Education Cloud data services to predict student success outcomes and make decisions about distributing financial aid and scholarships (Pangburn, 2019).
Understandably, admissions officials want to admit students who have the highest likelihood of “succeeding” (i.e. persisting through to graduation). Noting that the Salesforce AI predictive tools somehow account for bias that may exist in raw data reporting (like “name coding” or zip code bias), companies with products similar to the Education Cloud market fairer, more objective, more scientific ways to predict student success (Koenig, 2020). As a result, HEIs like Taylor are confidently using these kinds of tools in the admissions process to help counteract biases that grow “situationally” and often unexpectedly from how admissions officers review applicants, including an inconsistent number of reviewers, reviewer exhaustion, personality preferences, etc. (Pangburn, 2019). Additionally, AI assists with more consistent and comprehensive “background” checks for student data reported on an application (e.g. confirming whether or not a student was really an athlete) (Pangburn, 2019). Findings from the Alvero et al (2020) study mentioned earlier suggested that AI use and data auditing might be useful in informing the review process by checking potential bias in human or computational readings.
Another interesting proposal for the use of tech in the admissions process is the gamification of data points. Companies like KnackApp are marketing recruitment tools that would have applicants play a game for 10 minutes. Behind the scenes, algorithms allegedly gather information about users’ “microbehaviors,” such as the types of mistakes they make, whether those mistakes are repeated, the extent to which the player takes experimental paths, how the player is processing information, and the player’s overall potential for learning (Koenig, 2020). The CEO of KnackApp, Guy Halftek, claims that colleges outside the U.S. already use KnackApp in student advising, and the hope is that U.S. colleges will begin using the platform in the admissions process to create gamified assessments that would provide additional data points and measurements for desirable traits that might not otherwise be found in standardized test scores, GPA, or an entrance essay (Koenig, 2020).
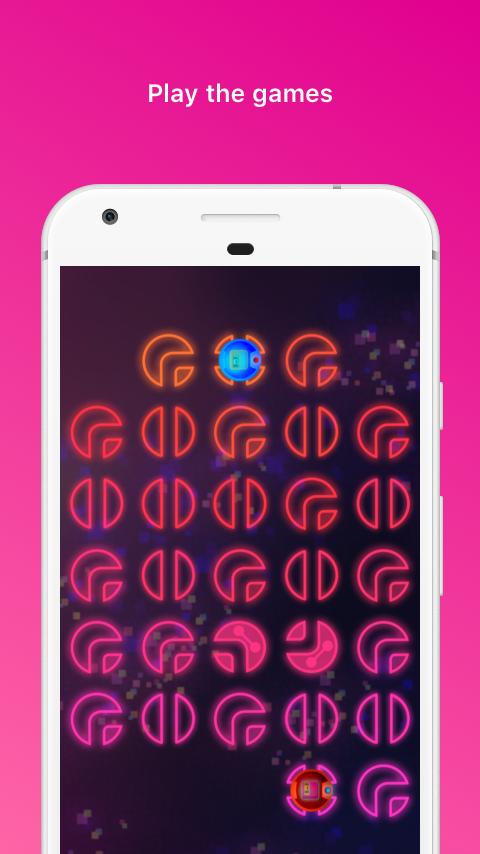
Regardless of its specific function in the overall process, AI and algorithms are being pitched as a way to make the admissions system more equitable by identifying authentic data points and helping schools reduce unseen human biases that can impact admissions decisions while simultaneously making bias pitfalls more explicit.
What’s The Catch?
Without denying the ways in which technology has offered significant assistance to—and perhaps progress in—the world of HEI admissions, it’s wise to think critically about the function of AI and algorithms and whether or not they are in fact assisting in a quest for equity.
To begin with, there is a persistent concern among digital ethicists that AI and algorithms simply mask and extend preexisting prejudice (Koenig, 2020). It is dangerous to assume that technology is inherently objective or neutral, since technology is still created or designed by a human with implicit (or explicit) bias (Benjamin, 2019). As Ruha Benjamin states in the 2019 publication Race After Technology: Abolitionist Tools for the New Jim Code, “…coded inequity makes it easier and faster to produce racist outcomes.” (p. 12)
Some areas of concern with using AI and algorithms in college admissions include:
- Large software companies like Salesforce seem to avoid admitting that bias could ever be an underlying issue, and instead seem to market that they’ve “solved” the bias issue (Pangburn, 2019).
- Predictive concerns: if future decisions are made on past data, a feedback loop of replicated bias might ensue (Pangburn, 2019).
- If, based on data, universities strategically market only to desirable candidates, they’ll likely pay more visits and make more marketing efforts to students in affluent areas and those who are likely to yield more tuition revenue (Pangburn, 2019).
- When it comes to “data-based” decision-making, it’s easier to get data for white, upper-middle-class suburban kids, and models (for recruiting goals, student success, and graduation outcomes) end up being built on easier data (Koenig, 2020).
- Opportunities for profit maximization are often rebranded as bias minimization, regardless of the extent to which that is accurate (Benjamin, 2019)
- Data privacy… (Koenig, 2020)
Finally, there’s always the question of human abilities and “soft skills,” and to what extent those should be modified or replaced by AI in any professional field. There’s no denying the limitations AI and algorithms face in making appropriate contextual considerations. For example, how does AI account for a high school or for-profit college that historically participates in grade inflation? How does AI account for additional challenges faced by a lower income or first-generation student? (Pangburn, 2019) There are also no guarantees that applicants won’t figure out how to “game” data-based admissions systems down the road by strategically optimizing their own data, and if/when that happens, you can bet that the most educated, wealthiest, highest-resourced students and families will be the ones optimizing that data, therefore replicating a system of bias and inequity that already exists (Pangburn, 2019).
As an admissions official at a small, liberal arts institution, I am well aware of the challenges presented to recruitment and admissions processes in the present and future, and am heartened to consider the possibilities that AI and algorithms might bring to the table, especially regarding efforts towards equitable admissions practices and recruiting more diverse student bodies. However, echoing the sentiments of Ruha Benjamin in The New Jim Code, I do not believe that technology is inherently neutral, and I do not believe that the use of AI or algorithms are comprehensive solutions for admissions bias. Higher education officials must proceed carefully, thoughtfully, and with the appropriate amount of skepticism.
References:
Alvero, A.J., Arthurs, N., Antonio, A., Domingue, B., Gebre-Medhin, B., Gieble, S., & Stevens, M. (2020). AI and holistic review: Informing human reading in college admissions from the proceedings of the AAAI/ACM Conference on AI, Ethics, and Society, 200–206. Association for Computing Machinery. https://doi.org/10.1145/3375627.3375871
Benjamin, R. (2019). Race after technology: Abolitionist tools for the New Jim Code. Polity.
Choi, Y.W. (2020, March 31). How to address racial bias in standardized testing. Next Gen Learning. https://www.nextgenlearning.org/articles/racial-bias-standardized-testing
Greenspan, R. (2019, May 15). Lori Loughlin and Felicity Huffman’s college admissions scandal remains ongoing. Here are the latest developments. Time. https://time.com/5549921/college-admissions-bribery-scandal/
Hassan, A. (2019, November 5). 5 takeaways from the Harvard admissions ruling. The New York Times. https://www.nytimes.com/2019/10/02/us/takeaways-harvard-ruling-admissions.html
Koenig, R. (2020, July 10). As colleges move away from the SAT, will algorithms step in? EdSurge. https://www.edsurge.com/news/2020-07-10-as-colleges-move-away-from-the-sat-will-admissions-algorithms-step-in
Mintz, S. (2020, July 13). Equity in college admissions. Inside Higher Ed. https://www.insidehighered.com/blogs/higher-ed-gamma/equity-college-admissions-0
Pangburn, D. (2019, May 17). Schools are using software to help pick who gets in. What could go wrong? Fast Company. https://www.fastcompany.com/90342596/schools-are-quietly-turning-to-ai-to-help-pick-who-gets-in-what-could-go-wrong